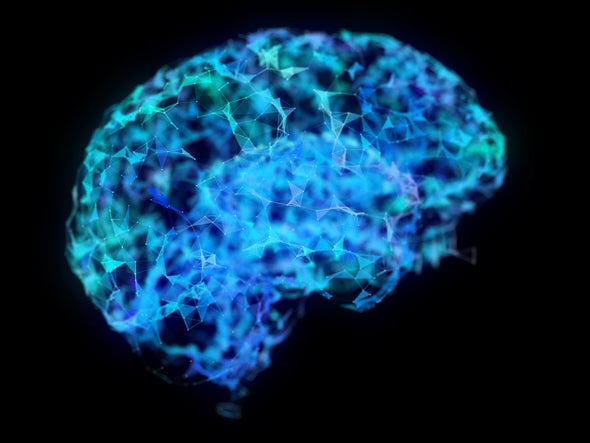
When Herbert Weinstein stood trial for the murder of his wife in 1992, his attorneys were struck by the measured calm with which he recounted her death and the events leading up to it. He made no attempt to deny that he was culpable, and yet his stoicism in the face of his wildly uncharacteristic actions led his defense to suspect that he might not be. Weinstein underwent neuroimaging tests, which confirmed what his attorneys had suspected: a cyst had impinged upon large parts of Weinstein’s frontal lobe, the seat of impulse control in the brain. On these grounds, they reasoned he should be found not guilty by reason of insanity, despite Weinstein’s free admission of guilt.
Guilt is difficult to define, but it pervades every aspect of our lives, whether we’re chastising ourselves for skipping a workout, or serving on the jury of a criminal trial. Humans seem to be hardwired for justice, but we’re also saddled with a curious compulsion to diagram our own emotional wiring. This drive to assign a neurochemical method to our madness has led to the generation of vast catalogs of neuroimaging studies that detail the neural underpinnings of everything from anxiety to nostalgia. In a recent study, researchers now claim to have moved us one step closer to knowing what a guilty brain looks like.
Since guilt carries different weight depending on context or culture, the authors of the study chose to define it operationally as the awareness of having harmed someone else. A series of functional magnetic resonance imaging (fMRI) experiments across two separate cohorts, one Swiss and one Chinese, revealed what they refer to as a “guilt-related brain signature” that persists across groups. Since pervasive guilt is a common feature in severe depression and PTSD, the authors suggest that a neural biomarker for guilt could offer more precise insight into these conditions and, potentially, their treatment. But brain-based biomarkers for complex human behaviors also lend themselves to the more ethically fraught discipline of neuroprediction, an emergent branch of behavioral science that combines neuroimaging data and machine learning to forecast how an individual is likely to act based on how their brain scans compare to those of other groups.
Predictive algorithms have already been used for years in health care, advertising and, most notoriously, the criminal justice system. Facial recognition and risk-assessment algorithms are criticized for their racial bias and tendency to be significantly less accurate when assigning offenders to “high risk” versus “low risk” categories. One of the highest profile exposures of such bias in recent news was a 2018 ACLU report on Amazon’s Rekognition algorithm for facial identification and analysis, which erroneously identified 28 members of Congress as criminal offenders when run against a database of mugshots. People of color made up almost 40 percent of the misidentified individuals, about double their portion of Congress. Amazon took vocal public issue with the methodology employed by the study at the time. However, just this summer they suspended the use of Rekognition by law enforcement for one year, amid a nationwide movement to dismantle the racially biased structures of policing and criminal justice that lead to the disproportionate death and incarceration of BIPOC.
Some researchers argue that neuroimaging data should theoretically eliminate the biases that emerge when predictive algorithms are trained on socioeconomic metrics and criminal records, based on the assumption that biological metrics are inherently more objective than other kinds of data. In one study, fMRI data from incarcerated people seeking treatment for substance abuse was fed through machine learning algorithms in an attempt to correlate activity in an area of the brain called the anterior cingulate cortex, or ACC, with the likelihood of completing a treatment program. The algorithm was able to correctly predict treatment outcomes about 80 percent of the time. Researchers have linked variations in ACC activity to violence, antisocial behavior and increased likelihood of rearrest in similar functional imaging studies. Indeed, the quest for the neural center of guilt in the brain also led to the ACC.
One of the problems with fMRI, though, is that it doesn’t directly measure neural firing patterns. Rather, it uses blood flow in the brain as a visual proxy for neural activity. Complex behaviors and emotional states engage multiple, widely distributed parts of the brain, and the patterns of activity within these networks provide more insight than viewing snapshots of activity in individual regions. So while it may be tempting for law enforcement to conclude that low ACC activity could be used as a biomarker for recidivism risk, altered ACC activation patterns are also hallmarks of schizophrenia and autism spectrum disorders. Rather than reducing bias by using presumably objective anatomical markers of neural activity, the use of behavioral biomarkers in a criminal justice context runs the risk of encouraging the criminalization of mental illness and neurodivergence.
There may be other limits to fMRI as a methodology. A recent large-scale review of numerous fMRI studies concluded that the variability of results, even at an individual level, is too high to meaningfully generalize them to larger groups, much less use them as the framework for predictive algorithms. The very notion of a risk assessment algorithm itself is based in the deterministic presupposition that people don’t change. Indeed, this determinism is characteristic of the retributive models of justice that these algorithms serve, which focus on punishing and incarcerating offenders, and not on addressing the conditions that led to an arrest in the first place.
Indeed, such use of brain imaging as a predictive tool in human behavior overlooks what seems to be a fundamental fact of neuroscience: that brains, like people, are capable of change; that they constantly remodel themselves, electrically and structurally, depending on experience. Rather than simply representing a more technologically complex means of meting out punishment, neuroprediction has the power to identify those same signatures and instead offer paths to intervention. Any algorithm, no matter how sophisticated, will always be as biased as the people who use it. We can’t begin to address these biases until we re-examine our basic approaches to criminality and justice.
"What" - Google News
November 18, 2020 at 07:00PM
https://ift.tt/3kH7DZB
What Does a Guilty Brain Look Like? - Scientific American
"What" - Google News
https://ift.tt/3aVokM1
https://ift.tt/2Wij67R
Bagikan Berita Ini
0 Response to "What Does a Guilty Brain Look Like? - Scientific American"
Post a Comment